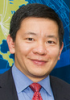
Large-scale environmental changes pose challenges that straddle environmental, economic, and social boundaries. As we design and implement climate adaptation strategies at the Federal, state, local, and tribal levels, accessible and usable data are essential for implementing actions that are informed by the best available information. Data-intensive science has been heralded as an enabler for scientific breakthroughs powered by advanced computing capabilities and interoperable data systems. Those same capabilities can be applied to data and information systems that facilitate the transformation of data into highly processed products.
At the interface of scientifically informed public policy and data intensive science lies the potential for producers of credible, integrated, multi-scalar environmental data like the National Ecological Observatory Network (NEON) and its partners to capitalize on data and informatics interoperability initiatives that enable the integration of environmental data from across credible data sources. NSF’s large-scale environmental observatories such as NEON and the Ocean Observatories Initiative (OOI) are designed to provide high-quality, long-term environmental data for research. These data are also meant to be repurposed for operational needs that like risk management, vulnerability assessments, resource management, and others. The proposed USDA Agriculture Research Service (ARS) Long Term Agro-ecosystem Research (LTAR) network is another example of such an environmental observatory that will produce credible data for environmental / agricultural forecasting and informing policy.
To facilitate data fusion across observatories, there is a growing call for observation systems to more closely coordinate and standardize how variables are measured. Together with observation standards, cyberinfrastructure standards enable the proliferation of an ecosystem of applications that utilize diverse, high-quality, credible data. Interoperability facilitates the integration of data from multiple credible sources of data, and enables the repurposing of data for use at different geographical scales. Metadata that captures the transformation of data into value-added products (“provenance”) lends reproducability and transparency to the entire process. This way, the datasets and model code used to create any product can be examined by other parties.